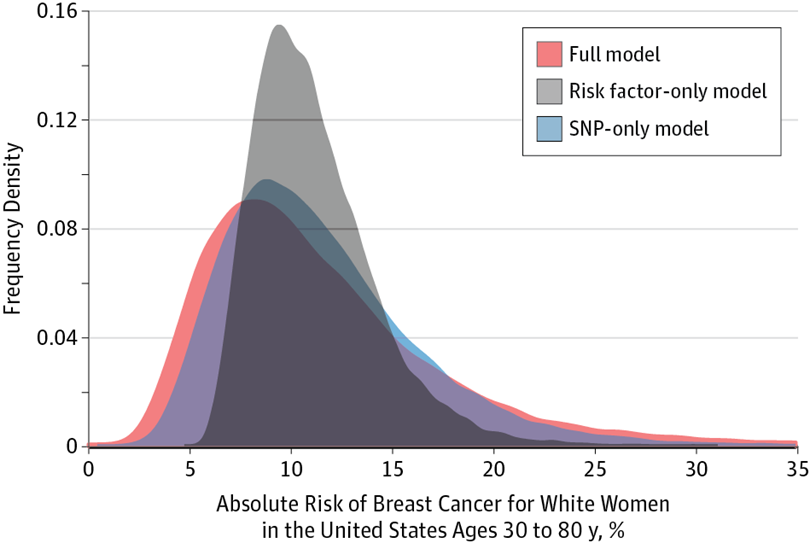
As we develop better understanding of disease risk factors, including genetic susceptibility, biomarkers, environmental and life-style factors, it is critical that such knowledge is translated for developing more effective strategies for disease prevention. A critical step towards this goal is to develop tools that can be used to predict future risk of diseases in healthy individuals and then tailor Disease risk prediction strategies for primary and secondary disease preventions based on individualized risks. Over several years, we have been developing methods for building, validating and assessing risk prediction models that can incorporate both genetic and non-genetic risk factors of diseases. We are studying powerful yet simple methods for constructing predictive polygenic risk score (PRS) using data from GWAS. Most recently, we have developed and distributed a software package, Individualized Coherent Absolute Risk Estimator (iCARE), for building models to predict absolute risks of diseases by synthesizing information from different data sources. We have used this methodology to develop specific models for risk prediction of breast cancer and have assessed clinical utility of such models.